⚡ Try out Aim at play.aimstack.io ⚡ |
---|
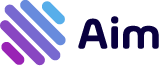
Aim logs your training runs, enables a beautiful UI to compare them and an API to query them programmatically.
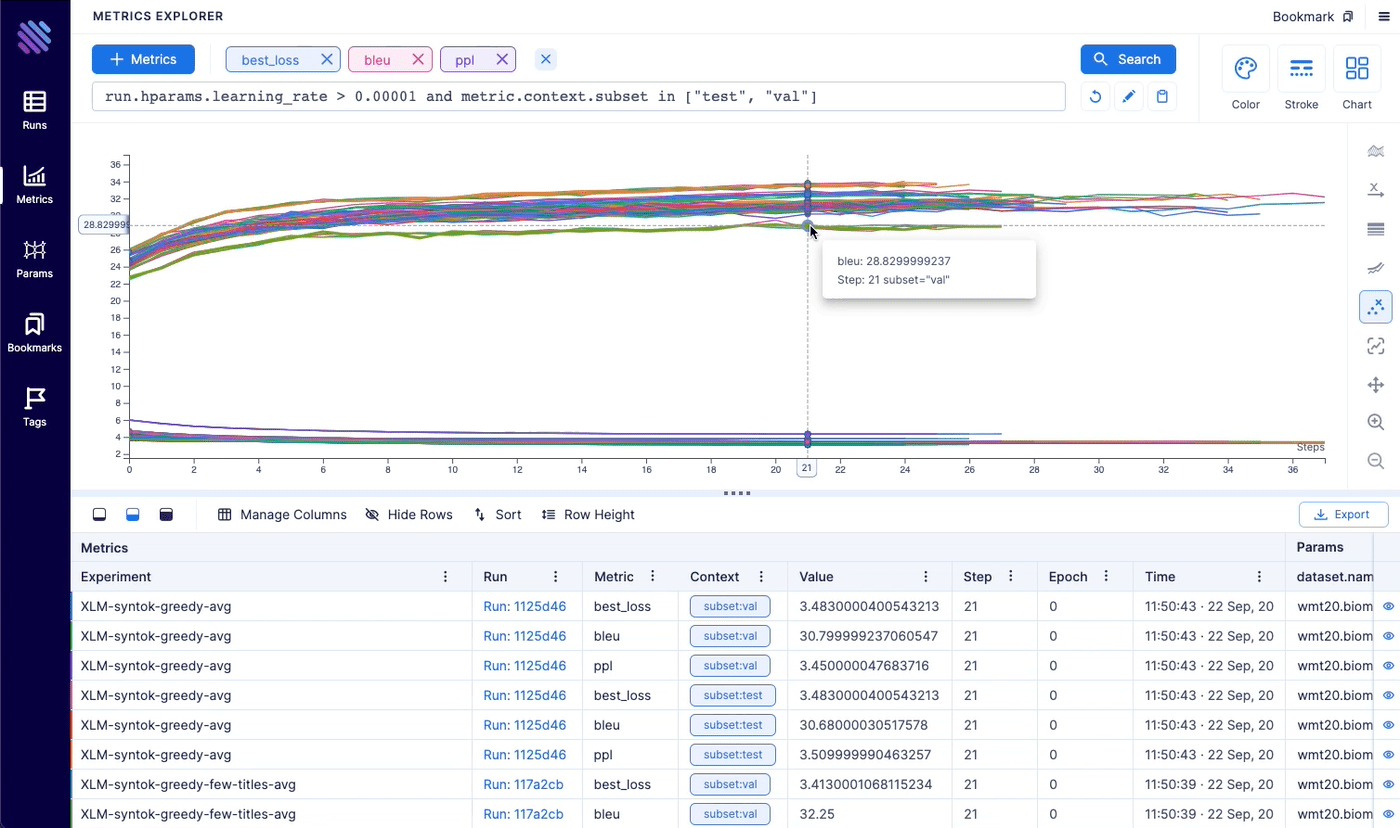
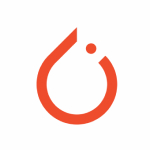
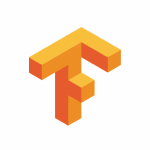
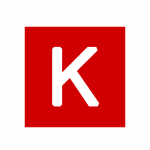
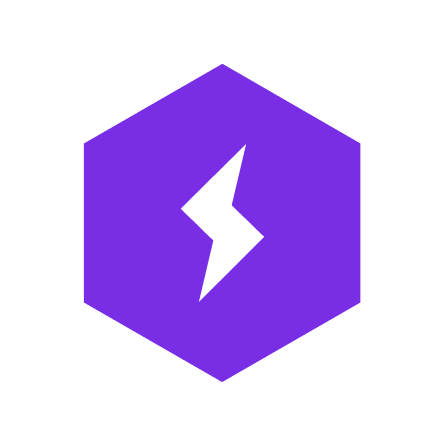
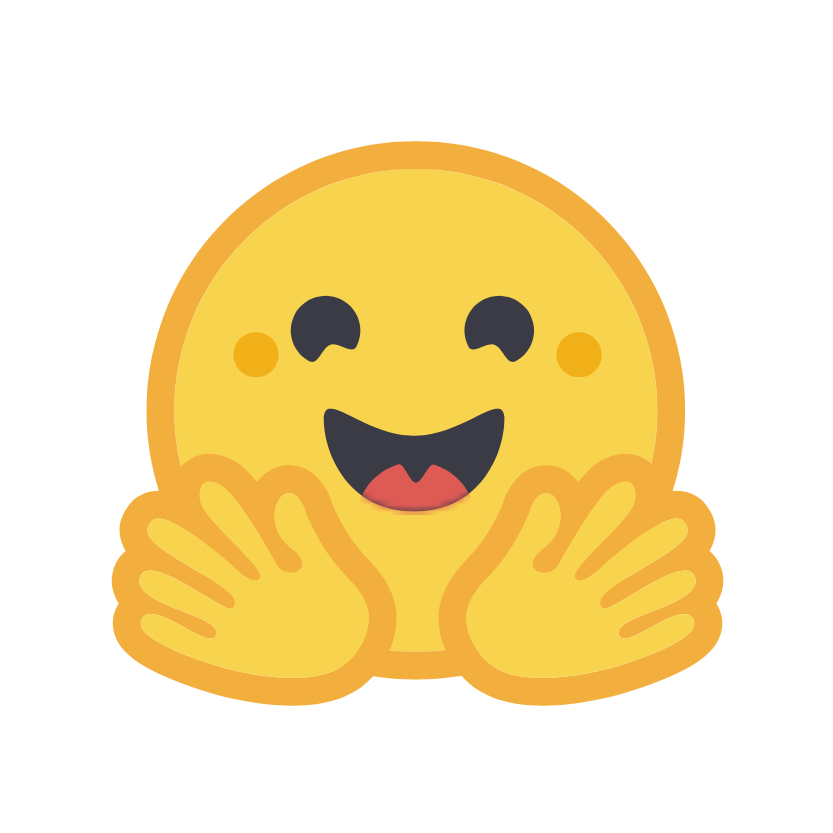
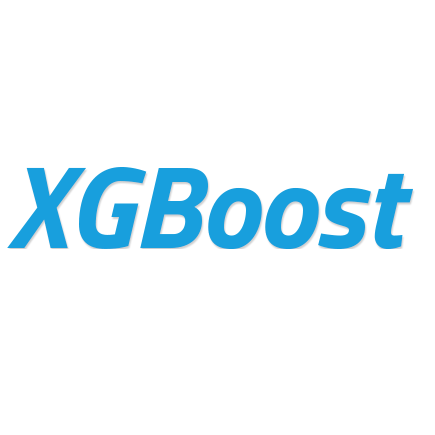
Aim is open-source, self-hosted AI experiment tracking tool. Use Aim to deeply inspect hundreds of hyperparameter-sensitive training runs at once.
Follow the steps below to get started with Aim.
1. Install Aim on your training environment
Prerequisite: You need to have python3 and pip3 installed in your environment before installing Aim
$ pip3 install aim
2. Integrate Aim with your code
Integrate your Python script
from aim import Run
# Initialize a new run
run = Run()
# Log run parameters
run["hparams"] = {
"learning_rate": 0.001,
"batch_size": 32,
}
# Log metrics
for step, sample in enumerate(train_loader):
# ...
run.track(loss_val, name='loss', step=step, epoch=epoch, context={ "subset": "train" })
run.track(acc_val, name='acc', step=step, epoch=epoch, context={ "subset": "train" })
# ...
See documentation here.
Integrate PyTorch Lightning
from aim.pytorch_lightning import AimLogger
# ...
trainer = pl.Trainer(logger=AimLogger(experiment='experiment_name'))
# ...
See documentation here.
Integrate Hugging Face
from aim.hugging_face import AimCallback
# ...
aim_callback = AimCallback(repo='/path/to/logs/dir', experiment='mnli')
trainer = Trainer(
model=model,
args=training_args,
train_dataset=train_dataset if training_args.do_train else None,
eval_dataset=eval_dataset if training_args.do_eval else None,
callbacks=[aim_callback],
# ...
)
# ...
See documentation here.
Integrate Keras & tf.keras
import aim
# ...
model.fit(x_train, y_train, epochs=epochs, callbacks=[
aim.keras.AimCallback(repo='/path/to/logs/dir', experiment='experiment_name')
# Use aim.tensorflow.AimCallback in case of tf.keras
aim.tensorflow.AimCallback(repo='/path/to/logs/dir', experiment='experiment_name')
])
# ...
See documentation here.
Integrate XGBoost
from aim.xgboost import AimCallback
# ...
aim_callback = AimCallback(repo='/path/to/logs/dir', experiment='experiment_name')
bst = xgb.train(param, xg_train, num_round, watchlist, callbacks=[aim_callback])
# ...
See documentation here.
3. Run the training as usual and start Aim UI
$ aim up
❇️ The Aim product roadmap
- The
Backlog
contains the issues we are going to choose from and prioritize weekly - The issues are mainly prioritized by the highly-requested features
The high-level features we are going to work on the next few months
Done
- Live updates (End: Oct 18 2021)
In progress:
- Images tracking and visualization (Start: Oct 18 2021)
- Centralized tracking server (Start: Oct 18 2021)
Track and Explore:
- Distributions tracking and visualization
- Transcripts tracking and visualization
- Runs side-by-side comparison
Data Backup:
- Cloud storage support: aws s3, gsc, azure storage
Reproducibility:
- Track git info, env vars, CLI arguments, dependencies
- Collect stdout, stderr logs
Integrations:
- Colab integration
- Jupyter integration
- plotly integration
- Kubeflow integration
- Streamlit integration
- Raytune integration
If you have questions please: