DIANNA is a Python package that brings explainable AI (XAI) to your research project. It wraps carefully selected XAI methods in a simple, uniform interface. It's built by, with and for (academic) researchers and research software engineers working on machine learning projects.
DIANNA software is addressing needs of both (X)AI researchers and mostly the various domains scientists who are using or will use AI models for their research without being experts in (X)AI. DIANNA is future-proof: one of the very few XAI library supporting the Open Neural Network Exchange (ONNX) format.
After studying the vast XAI landscape we have made choices in the parts of the XAI Taxonomy on which methods, data modalities and problems types to focus. Our choices, based on the largest usage in scientific literature, are shown graphically in the XAI taxonomy below:

The key points of DIANNA:
- Provides an easy-to-use interface for non (X)AI experts
- Implements well-known XAI methods LIME, RISE and KernelSHAP, chosen by systematic and objective evaluation criteria
- Supports the de-facto standard of neural network models - ONNX
- Supports images, text, time series, and tabular data modalities, embeddings are currently being developed
- Comes with simple intuitive image, text, time series, and tabular benchmarks, so can help you with your XAI research
- Includes scientific use-cases tutorials
- Easily extendable to other XAI methods
For more information on the unique strengths of DIANNA with comparison to other tools, please see the context landscape.
DIANNA can be installed from PyPI using pip on any of the supported Python versions (see badge):
python3 -m pip install dianna
To install the most recent development version directly from the GitHub repository run:
python3 -m pip install git+https://github.com/dianna-ai/dianna.git
If you get an error related to OpenMP when importing dianna, have a look at this issue for possible workarounds.
- To install TensorFlow you can follow this tutorial.
- To install TensorFlow Addons you can follow these steps. For further reading see this issue. Note that this temporary solution works only for macOS versions >= 12.0. Note that this step may have changed already, see #245.
- Before installing DIANNA, comment
tensorflow
requirement insetup.cfg
file (tensorflow package for M1 is calledtensorflow-macos
).
You need:
- your trained ONNX model (convert my pytorch/tensorflow/keras/scikit-learn model to ONNX)
- a data item to be explained
You get:
- a relevance map overlayed over the data item
- Provide your trained model and data item ( text, image, time series or tabular )
model_path = 'your_model.onnx' # model trained on your data modality
data_item = <data_item> # data item for which the model's prediction needs to be explained
- If the task is classification: which are the classes your model has been trained for?
labels = [class_a, class_b] # example of binary classification labels
Which of these classes do you want an explanation for?
explained_class_index = labels.index(<explained_class>) # explained_class can be any of the labels
- Run dianna with the explainer of your choice ( 'LIME', 'RISE' or 'KernalSHAP') and visualize the output:
explanation = dianna.<explanation_function>(model_path, data_item, explainer)
dianna.visualization.<visualization_function>(explanation[explained_class_index], data_item)
Lets illustrate the template above with textual data. The data item of interest is a sentence being (a part of) a movie review and the model has been trained to classify reviews into positive and negative sentiment classes. We are intersted which words are contributing positively (red) and which - negatively (blue) towards the model's desicion to classify the review as positive and we would like to use the LIME explainer:
model_path = 'your_text_model.onnx'
# also define a model runner here (details in dedicated notebook)
review = 'The movie started great but the ending is boring and unoriginal.'
labels = ["negative", "positive"]
explained_class_index = labels.index("positive")
explanation = dianna.explain_text(model_path, text, 'LIME')
dianna.visualization.highlight_text(explanation[explained_class_index], model_runner.tokenizer.tokenize(review))
Here is another illustration on how to use dianna to explain which parts of a bee image contributied positively (red) or negativey (blue) towards a classifying the image as a 'bee' using RISE.
The Imagenet model has been trained to distinguish between 1000 classes (specified in labels
).
For images, which are data of higher dimention compared to text, there are also some specifics to consider:
model_path = 'your_image_model.onnx'
image = PIL.Image.open('your_bee_image.jpeg')
axis_labels = {2: 'channels'}
explained_class_index = labels.index('bee')
explanation = dianna.explain_image(model_path, image, 'RISE', axis_labels=axis_labels, labels=labels)
dianna.visualization.plot_image(explanation[explained_class_index], utils.img_to_array(image)/255., heatmap_cmap='bwr')
plt.show()

And why would Imagenet think the same image would be a garden spider?
explained_class_index = labels.index('garden_spider') # interested in the image being classified as a garden spider
explanation = dianna.explain_image(model_path, image, 'RISE', axis_labels=axis_labels, labels=labels)
dianna.visualization.plot_image(explanation[explained_class_index], utils.img_to_array(image)/255., heatmap_cmap='bwr')
plt.show()

There are full working examples on how to use the supported explainers and how to use dianna for all supported data modalities in our overview tutorial.
The explainers are sensitive to the choice of their hyperparameters! In this work, this sensitivity to hyperparameters is researched and useful conclusions are drawn. The default hyperparameters used in DIANNA for each explainer as well as the values for our tutorial examples are given in the Tutorials README.
Explore the explanations of your trained model using the DIANNA dashboard (for now images, text and time series classification is supported). Click here for more information.
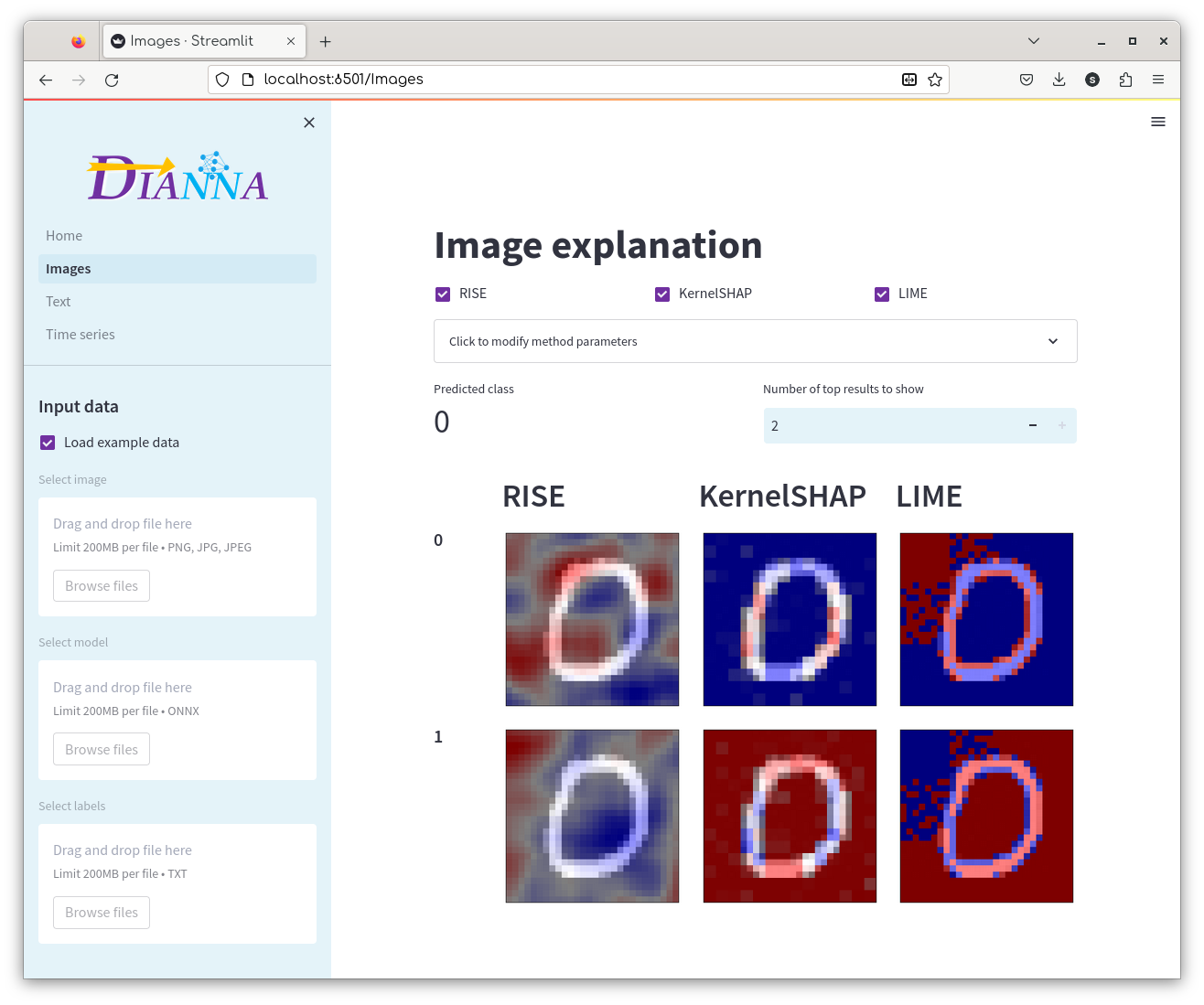
DIANNA comes with simple datasets. Their main goal is to provide intuitive insight into the working of the XAI methods. They can be used as benchmarks for evaluation and comparison of existing and new XAI methods.
Dataset | Description | Examples | Generation |
---|---|---|---|
Binary MNIST ![]() |
Greyscale images of the digits "1" and "0" - a 2-class subset from the famousMNIST dataset for handwritten digit classification. | ![]() |
Binary MNIST dataset generation |
Simple Geometric (circles and triangles) ![]() |
Images of circles and triangles for 2-class geometric shape classificaiton. The shapes of varying size and orientation and the background have varying uniform gray levels. | ![]() |
Simple geometric shapes dataset generation |
Simple Scientific (LeafSnap30) ![]() |
Color images of tree leaves - a 30-class post-processed subset from the LeafSnap dataset for automatic identification of North American tree species. | ![]() |
LeafSnap30 dataset generation |
Dataset | Description | Examples | Generation |
---|---|---|---|
Stanford sentiment treebank ![]() |
Dataset for predicting the sentiment, positive or negative, of movie reviews. | This movie was actually neither that funny, nor super witty. | Sentiment treebank |
Dataset | Description | Examples | Generation |
---|---|---|---|
Coffee dataset |
Food spectographs time series dataset for a two class problem to distinguish between Robusta and Arabica coffee beans. | data source | |
Weather dataset |
The light version of the weather prediciton dataset, which contains daily observations (89 features) for 11 European locations through the years 2000 to 2010. | data source |
Dataset | Description | Examples | Generation |
---|---|---|---|
Pengiun dataset |
Palmer Archipelago (Antarctica) penguin dataset is a great intro dataset for data exploration & visualization similar to the famous Iris dataset. | ![]() |
data source |
Weather dataset |
The light version of the weather prediciton dataset, which contains daily observations (89 features) for 11 European locations through the years 2000 to 2010. | data source |
We work with ONNX! ONNX is a great unified neural network standard which can be used to boost reproducible science. Using ONNX for your model also gives you a boost in performance! In case your models are still in another popular DNN (deep neural network) format, here are some simple recipes to convert them:
- pytorch and pytorch-lightning - use the built-in
torch.onnx.export
function to convert pytorch models to onnx, or call the built-into_onnx
function on yourLightningModule
to export pytorch-lightning models to onnx. - tensorflow - use the
tf2onnx
package to convert tensorflow models to onnx. - keras - same as the conversion from tensorflow to onnx, the
tf2onnx
package also supports keras. - scikit-learn - use the
skl2onnx
package to scikit-learn models to onnx.
More converters with examples and tutorials can be found on the ONNX tutorial page.
And here are links to notebooks showing how we created our models on the benchmark datasets:
Models | Generation |
---|---|
Binary MNIST model | Binary MNIST model generation |
Simple Geometric model | Simple geometric shapes model generation |
Simple Scientific model | LeafSnap30 model generation |
Models | Generation |
---|---|
Movie reviews model | Stanford sentiment treebank model generation |
Models | Generation |
---|---|
Penguin model (classification) | Penguin model generation |
Sunshine hours prediction model (regression) | Sunshine hours prediction model generation |
We envision the birth of the ONNX Scientific models zoo soon...
DIANNA supports different data modalities and XAI methods (explainers). We have evaluated many explainers using objective criteria (see the How to find your AI explainer blog-post). The table below contains links to the relevant XAI method's papers (for some explanatory videos on the methods, please see tutorials). The DIANNA tutorials cover each supported method and data modality on a least one dataset using the default or tuned hyperparameters. Our plans to expand DIANNA with more data modalities and explainers are given in the ROADMAP.
Data \ XAI | RISE | LIME | KernelSHAP |
---|---|---|---|
Images | โ | โ | โ |
Text | โ | โ | |
Timeseries | โ | โ | |
Tabular | planned | โ | โ |
Embedding | work in progress | ||
Graphs* | next steps | ... | ... |
LRP and PatternAttribution also feature in the top 5 of our thoroughly evaluated explainers. Also GradCAM) has been recently found to be semantically continous! Contributing by adding these and more (new) post-hoc explainability methods on ONNX models is very welcome!
Our goal is that the scientific community embrases XAI as a source for novel and unexplored perspectives on scientific problems. Here, we offer tutorials on specific scientific use-cases of uisng XAI:
Use-case (data) \ XAI | RISE | LIME | KernelSHAP |
---|---|---|---|
Biology (Phytomorphology): Tree Leaves classification (images) | โ | ||
Astronomy: Fast Radio Burst detection (timeseries) | โ | ||
Geo-science (raster data) | planned | ... | ... |
Social sciences (text) | work in progress | ... | ... |
Climate | planned | ... | ... |
For detailed information on using specific DIANNA functions, please visit the documentation page hosted at Readthedocs.
If you want to contribute to the development of DIANNA, have a look at the contribution guidelines. See our developer documentation for information on developer installation, running tests, generating documentation, versioning and making a release.
If you use this package for your scientific work, please consider citing directly the software as:
Ranguelova, E., Bos, P., Liu, Y., Meijer, C., Oostrum, L., Crocioni, G., Ootes, L., Chandramouli, P., Jansen, A., Smeets, S. (2023). dianna (*[VERSION YOU USED]*). Zenodo. https://zenodo.org/record/5592606
or the JOSS paper as:
Ranguelova et al., (2022). DIANNA: Deep Insight And Neural Network Analysis. Journal of Open Source Software, 7(80), 4493, https://doi.org/10.21105/joss.04493
See also the Zenodo page or the JOSS page for exporting the software citation to BibTteX and other formats.
This package was created with Cookiecutter and the NLeSC/python-template.