I have a dataset where the number of components with any criterion were sometimes way to high or low. This is an issue that has been reported by others. I've identified one reason this is happening.
The MAPCA algorithm depends on estimating the spatial dependence of voxels and then running a cost function on a sparse sampling of the voxels that are far enough apart to be independent & identically distributed (i.i.d.). That is, if neighboring voxels have dependence, then apply the cost function to every other voxel in 3D space (i.e. use 1/(2^3) of the total voxels).
I made a branch of mapca which outputted a lot more logging including this subsampling factor: sub_iid_sp_median
The attached figure shows this subsampling factor on the x axis and the estimated number of components on the y axis. The different markers are different run types, but all have 300-350 volumes. When the subsampling factor is 1 (i.e. use every voxel) the number of components estimation always fails by giving way too many components. When the subsampling factor is 3 (1/27 of the total voxels used for estimation) the number of components estimation fails by being way too low. I'm Given the very large range of estimated components when the subsampling factor is 2, I suspect there are other issues, but this discrete parameter should be constant for data collected within the same acquisition parameters and bad things happen when it's not.
I'm starting to think about options for completely different approaches for estimating the number of components, but I wanted to document this here. As an intermediate step, we might want to add a few more values, such as this subsamplign factor, into logging.
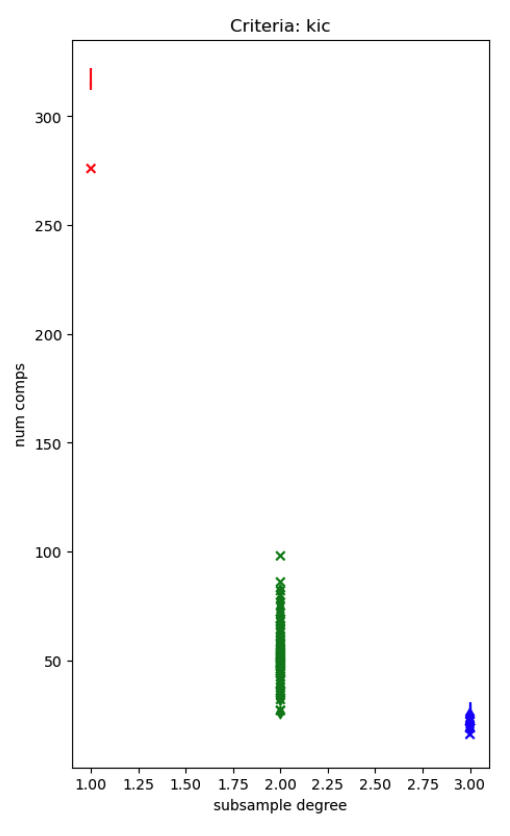
As a tangent, I realized mapca used a LGR
but it wasn't writing out the log. In my above branch, I changed the LGR declaration to "general" and changed a few other things. MAPCA still didn't output it's own log, but then the LGR output from mapca was included in the tedana log.
https://github.com/handwerkerd/mapca/blob/a03727b3346c0f92380b5a1699b7c001d43c6956/mapca/mapca.py#L31